In its simplest form, digital manufacturing uses electronic information from or related to manufacturing operations to support more informed decision-making and increase the efficiency of personnel, equipment, and processes.
That decision-making process may be as basic as using data from a production report to influence your next decisions, as complex as a fully automated “lights-out” production operation, or anything in between. The best solution is the one that fulfills the specific needs of your business.
A major challenge facing business managers is their dependency on the quality of the information provided. Is that information accurate? Does it properly represent the information that it is intended to represent? All too often, information can be skewed (intentionally or unintentionally) to represent a desired outcome. Decisions made based on faulty information typically are also equally flawed. It is tantamount that those responsible for business decisions fully understand the quality and viability of the information they are consuming or automated processes in making decisions.
Throughout manufacturing, we deploy lots of technology. And that technology, be it equipment or software systems, can provide valuable information to perform its intended task. Typically, we take such information at face value, often without a good understanding of the validity of that information. This information typically feeds into higher-level decision processes. If any information is flawed or misleading, then any higher-level decisions based on that information are tainted. The proverbial “garbage in, garbage out.”
Any decision process, be it an automated process on the shop floor or a decision in the board room, can be represented by a common simple model:


Information is consumed; it is processed through a set of rules or assumptions (analytics), and a set of outputs are generated. Two fundamental portions of this model impact the validity of the results – the quality of the inputs and the validity of the analytics. Flaws in either of these produce invalid results.
Within digital manufacturing, we are not dealing with a single decision process. Digital manufacturing is an integrated set of distributed decision processes that form an overall implementation – a “decision tree.” Any one piece of faulty or misapplied information, or the results of a distorted analytic calculation, can cascade bad information throughout the balance of the decision tree.
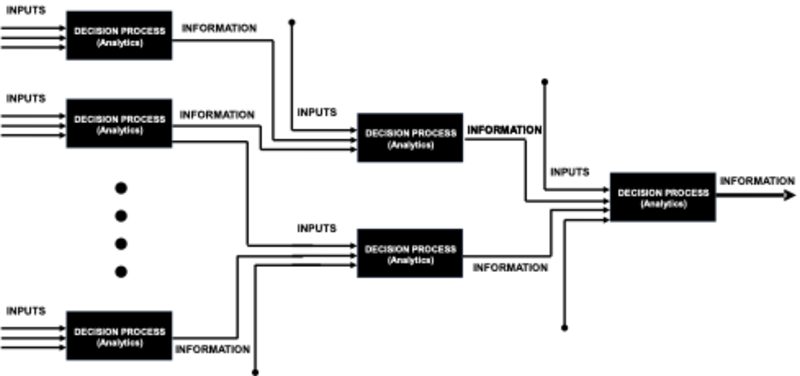
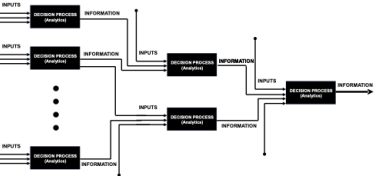
Determining the quality of inputs to the decision process varies depending on where those inputs are produced in a digital manufacturing operation. At the lower levels on the shop floor, most of the inputs are generated from sensors or pieces of equipment. Generally, this raw data is pretty reliable as long as there is some validity testing on that data and the equipment is properly maintained and calibrated. The area of greater concern is when raw data is processed through analytics, and the results then become the inputs to a higher-level decision process. It is important to know how that data has been manipulated. Higher in the decision tree, data from diverse sources may be introduced. It is incumbent on system implementors and business managers to qualify such data for both validity and applicability to its intended use.
Analytics is the portion of each decision process where most faulty information is generated. Two factors in each analytic process specifically impact the eventual results – the calculation applied to the data and the configuration parameters applied to those calculations.
For those decision processes that reside on the shop floor and those somewhat higher-level systems reporting information about shop operations, the calculation portion of the analytics is typically supplied by the vendors of the products deployed – either piece of equipment or software systems. All of these produce information that feeds into higher-level decision processes. Most business managers assumethat the information from these systems is valid – and never ask, “How are the outputs being calculated?” or “What does the output represent?” A simple example of where answers to those questions are important would be the case where a piece of equipment provides data on “hours of operation.” For that piece of equipment, does the data represent the hours the equipment was powered, or the hours the equipment produced work productively? This is key information needed before data produced by that piece of equipment is fed into a higher-level decision process reporting on equipment availability or utilization. Such an examination should be applied to every piece of data utilized in the decision tree.
The configuration parameters applied to an analytic calculation are the area where greater variability can be introduced in any decision process. For example, two identical pieces of software configured by two different individuals can produce significantly different results. Each individual’s perspective of best representing the information to achieve the desired results will influence the configuration process. For instance, one software system configured from maintenance’s perspective for reporting machine availability will likely result in significantly different results than the same software system configured from production’s perspective – different results generated from identical analytics but configured differently. Misapplication of configuration parameters has produced machine utilization values and OEE calculations with values greater than 100%, which are technically impossible. It is critical to truly understand the validity of data produced from analytics before consuming this information as input to a higher-level decision process.
Data and analytics are essential components of every digital manufacturing implementation. Business managers must dig deep into each decision point to (1) verify the validity of data used in every level of the digital decision tree and (2) validate that the analytics used at each decision point produces the intended results. The validity of data produced from vendor equipment should not be taken for granted. It is acceptable to challenge equipment and software system suppliers to identify the specifics of the analytic calculations used in their products. If they are unwilling to provide these details, it may be time to look for a different supplier. As shown, the configuration parameters applied to analytics can greatly impact the output results. Ensuring that software systems are configured to produce the results that best represent the specific manufacturing process is important.
You can learn more details about deploying a modular digital manufacturing information system in AMT’s “Digital Manufacturing” white paper series: “Overview Of Digital Manufacturing: Data-Enabled Decision-Making.”